Members of Bench-to-Bedside e/MTIC team from Catharina Hospital (CZE) and Philips Research in Eindhoven, the Netherlands, got two presentations accepted at the Predictive Analytics World in Healthcare Conference to be held June 21-23, 2022 in Las Vegas, USA. The Bench-to-Bedside team strives to bring AI from research (bench) to bedside implementation.
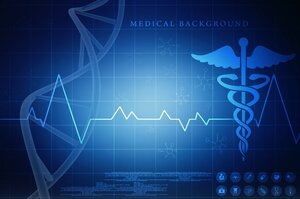
鈥淕iven the capacity challenges we face now and in the years ahead, a focus on efficient use of our OR, ICU and ward resources will be vital to provide the timely care our patients need. The increased demand for care, partially delayed due to the COVID-19 pandemic, requires optimization of the planning and use of resources,鈥 says Rikkert Keldermann, capacity manager at CZE. 鈥淭hese challenges can benefit of AI-driven approach that unlocks the power of data and provides true, actionable insights.鈥 For example, 鈥淭imely planning and predicting the bed type (PACU/ICU) that a patient will need after a cardiothoracic surgery relies on anesthesiologist鈥檚 clinical decision,鈥 clarifies Arthur Bouwman, anesthesiologist at CZE. 鈥淎 predictive model as a second opinion might be beneficial for accurate recommendations and support clinical decision making.鈥
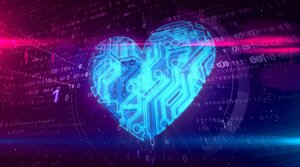
As a part of the conference agenda, the team will share recent results on data-driven capacity management for cardiothoracic surgery. 鈥淲e used data from 3,000 cardiothoracic surgeries to develop and validate predictive models of surgery duration and ICU-bed type鈥, said Mariana Simons and Marko Djikic, Senior Data Scientists at Philips Research. The ensembled predictive model of acute cardiothoracic surgery duration reduced the number of surgeries 鈥渂ehind-the-schedule鈥 by 28% (from 60% to 32%) and boosted the surgery 鈥渙n-time鈥 by 15% (from 30% to 45%). A hybrid model of ICU-bed type that combines the anesthesiologist鈥檚 recommendation with machine learning model鈥檚 predictions was able to reduce the misclassifications of PACU instead of ICU bed from the current 1-in-5 to 1-in-10.
Using machine learning technologies, based on specific individual patient characteristics, allows the creation of customized models for optimizing the OR and ICU utilization. Surgery planners could benefit from these predictive models by creating an optimized surgery schedule as a prerequisite to effective use of capacity management and improved patient and staff satisfaction.
For further information and . You can contact us via: bench2bedside@ emtic.nl