Researcher in the Spotlight: Robin de Rozario
I identify and control position-dependent mechanical systems
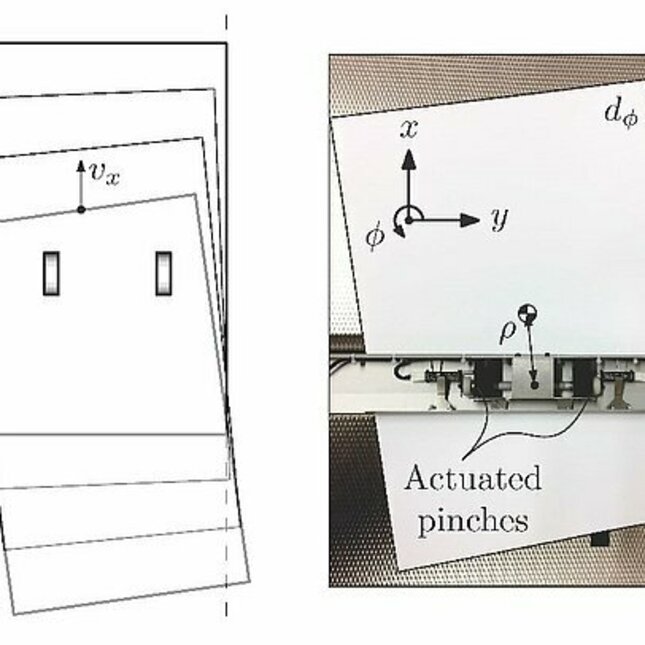
"Hi, my name is Robin de Rozario and I鈥檓 a PhD student at the research group Control Systems Technology of the department Mechanical Engineering. My research concerns the identification and control of position-dependent mechanical systems for large printer manufacturer Oc茅 Technologies. Maarten Steinbuch is my promotor at 果冻传媒."
"Increasing accuracy and throughput requirements on the next-generation manufacturing machines are creating a situation in which these systems can no longer be accurately controlled using controllers designed using classical approaches. One of the key reasons is that these complex mechatronic systems display strong position-dependent variations in their dynamics during operation and current control strategies are insufficiently developed to handle these variations appropriately. Consider, for example, the sheet position correction unit shown in Figure 1. Due to the constant velocity of the sheets, the effective inertia that the control systems 鈥渇eels鈥 changes rapidly, which makes it difficult to accurately control the position of the sheet."
Extend current control techniques
"The aim of this project is to extend the current control techniques to enable high performance for position-dependent mechatronic systems. Since system data is typically inexpensive and fast to obtain, this project focuses on using learning and identification techniques to achieve high performance and to estimate accurate models for subsequent optimal control design."
Design learning filters
"In this work, it is assumed that the variations in (linear time-invariant) dynamics can be directly related to a measureable quantity, which is referred to as the scheduling parameter, e.g., the relative position of the individual parts of the machine. The control theory that governs with this type of system is known as the Linear Parameter Varying (LPV) framework. The approach taken in this project is to extend the LPV framework to identify both non-parametric and parametric models and to generalize the Iterative Learning Control (ILC) method to design learning filters for LPV systems using both types of models or by directly exploiting the measured data."
Develop framework for user-friendly methodologies
"The main challenge of this work is to develop a framework of user-friendly methodologies with intuitive design guidelines for both controller/learning filter design and identification experiment design. A key practical challenge is to assess the effectiveness of the LPV approach and to provide insight in when the developed techniques necessary for the desired level of performance. On the theoretical side, challenges with respect to LPV controller synthesis include large convex optimization problems, conservatism of existing techniques in terms of guarantees on performance and stability, controller structure selection and possible non-causal dependence on the scheduling parameter. As for identification, the challenges include frequency domain input-output coupling, model structure selection and the curse of dimensionality due to the increased number of model parameters."
Significant progress in control
"In terms of control, significant progress is made in terms of generalizing the infinite time ILC framework and the ILC with basic functions framework towards LPV systems. In addition, novel data-driven iterative control methods have been developed for Linear Time-Invariant (LTI) systems and an extension towards LPV systems is currently being developed. In terms of identification techniques, various techniques to estimate both nonparametric and nonparametric models of mechanical systems are currently being developed, with promising initial results."
Extend toolbox of systems
"This research has the potential to significantly extend the 鈥渢oolbox鈥 of the systems and control engineer thereby enabling high-performance control and accurate identification of increasingly complex parameter-varying systems. This, in turn, leads to performance enhancement of existing and future systems that benefit from learning such as (3D) printing systems, (medical) robotics, lithographic systems and microscopy systems."
Collaboration
"Together with fellow PhD student Lennart Blanken, also part of the 果冻传媒 Control Systems Technology research group, we are doing research on 鈥楥ontrolling of Printing Systems鈥. While I鈥檓 focusing on 鈥業dentification and control of position-dependent mechanical systems鈥, Lennart鈥檚 research is to gain repetitive and learning motion control in printing systems. We discuss new ideas on an abstract level, attend scientific conferences together and we both visit Oc茅 Technologies about once a week where we supervise our MSc students. Furthermore, a quarterly progress meeting is held at Oc茅 where we discuss the results achieved and the current projects with company staff and our 果冻传媒 supervisors."